Data Science: The Driving Force Behind the Digital Age
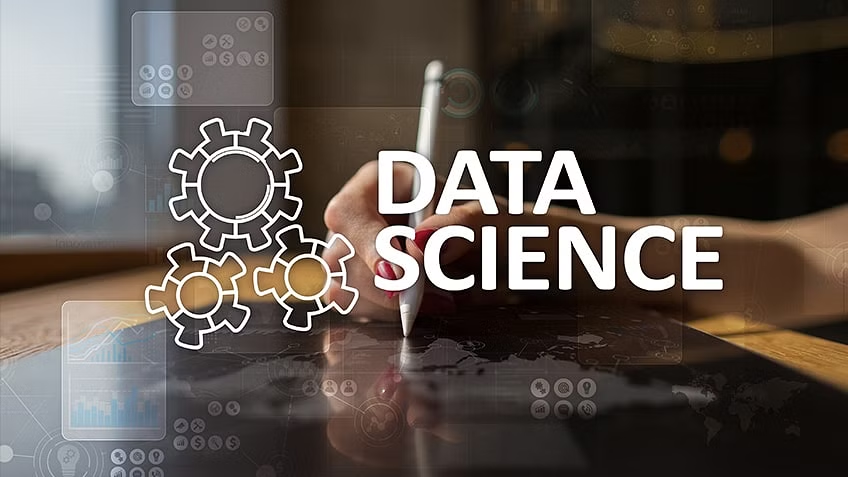
Data science has emerged as a transformative force in the modern world, shaping industries from finance to healthcare. At its core, data science is a blend of mathematics, statistics, computer science, and domain expertise that helps organizations make sense of complex data.
What is Data Science?
Data science refers to the use of algorithms, machine learning models, and other tools to extract knowledge and insights from structured and unstructured data. It combines techniques from multiple disciplines to process massive data sets and generate actionable insights.
Importance of Data Science in Today’s World
Data has become the backbone of the digital era. From social media platforms collecting user data to financial institutions analyzing transaction patterns, data science enables businesses to understand trends, predict future outcomes, and make data-driven decisions. In industries such as retail, data science helps companies personalize customer experiences, while in healthcare, it aids in diagnosing diseases and predicting patient outcomes.
Key Use Cases in Data Science
- E-commerce Personalization: Companies like Amazon and Netflix use data science algorithms to recommend products and shows based on user behavior.
- Healthcare: Data science plays a critical role in precision medicine, where algorithms predict treatment outcomes and personalize healthcare plans.
- Finance: Banks and financial institutions use data science for fraud detection and risk management.
Challenges in Data Science
Despite its wide-reaching benefits, data science faces several challenges. One major issue is dealing with unstructured data, such as text and images, which are harder to analyze than traditional structured data. Another challenge is ensuring data privacy and security, especially when working with sensitive information.
Future Trends in Data Science
As the field matures, several trends are set to shape its future:
- Automated Machine Learning (AutoML): Tools that automate the machine learning pipeline, making data science more accessible to non-experts.
- Edge Computing: Real-time data analysis on devices closer to the data source, reducing latency and bandwidth.
- Data Democratization: Tools and platforms that empower non-technical users to access and analyze data without needing in-depth coding skills.